- Accounting essay 代写
- America Essay 代写
- Anthropology essay 代写
- Architecture Essay代写
- Arts essay 代写
- Biology Essay 代写
- Business Essay 代写
- Chemistry essay代写
- Commerce Essay 代写
- Economics Assignment 代写
- Communications Essay 代写
- Computer Science Essay 代写
- Construction Essay 代写
- Criminology Essay 代写
- Cultural Studies Essay 代写
- Economics Essay 代写
- Education Essay 代写
- Engineering Essay 代写
- English Literature Essay 代写
- Environmental Sciences 代写
- Film Studies Essay 代写
- Finance Essay 代写
- General Studies Essay 代写
- Health Essay 代写
- History Essay 代写
- Human Resource Essay 代写
- Information Technology 代写
- It Research Essay 代写
- Law Essay 代写
- Management Essay 代写
- Marketing Essay 代写
- Media Essay 代写
- Nursing Essay 代写
- Philosophy Essay 代写
- Physical Education Essay 代写
- Make My Essay
- Politics Essay代写
- Psychology Essay 代写
- Religion Essay 代写
- Sciences Essay 代写
- Social Work Essay 代写
- Sociology Essay 代写
- Theology Essay 代写
- Tourism Essay 代写
- Weekly Studies 代写
- Dissertation 代写
- Coursework 代写
- APA Essay 代写
- MHRA essay 代写
- MBA Essay 代写
- Homework 代写
- Research Paper 代写
- 国立大学代写Essay
- 昆士兰代写ESSAY
- 墨尔本Melbourne代写Essay
- Monash 莫纳什 essay 代写
- Sydney悉尼代写essay
- 阿德莱德Adelaide代写Essay
- Brisbane布里斯班代写Essay
- 西澳代写essay
- Macquarie麦考瑞代写essay
- Deakin 迪肯代写Essay
- 南澳代写essay
- 纽卡斯尔代写Essay
- 塔斯马尼亚代写Essay
- Wollongong 卧龙岗代写Essay
- Griffith格里菲斯代写Essay
- LaTrobe拉筹伯代写Essay
- HR Essay 代写
- Perth珀斯代写Essay
- 詹姆斯库克代写essay
- Murdoch莫道克代写Essay
- 埃迪斯科文代写Essay
- Wollongong卧龙岗代写assignment
- Charles查尔斯特代写Essay
- MYOB paper 代写
- 维多利亚代写Essay
- 斯文本代写essay
- SPSS paper 代写
- 堪培拉代写Essay
- 英国代写essay
- 邦德代写Essay
- 南十字星代写essay
- 阳光海安代写Essay
- 查尔斯达尔文代写Essay
- Wellington 惠灵顿 Essay 代写
- Massey 梅西大学代写Essay
- Thesis Paper 代写
- Auckland奥克兰代写Essay
- Otago奥塔哥代写essay
堪培拉Essay代写:研究人员
2017-02-15 12:55
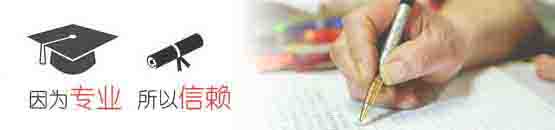
堪培拉Essay代写:研究人员
In the past decades, due to the unavailability of the software for fitting multivariate multilevel data some researchers tend to use manual methods such as EM Algorithm (Kang et al., 1991). As a result of developing the technical environment, the software such as STATA, SAS and S plus are emerged in to the Statistical field by providing facilitates to handle the multilevel data. But none of those packages have a capability of fitting multivariate multilevel data. However there is evidence in the literature that nonlinear multivariate multilevel model can be fitted using packages such as GLLAMM (Rabe-Hesketh, Pickles and Skrondal, 2001) and aML (Lillard and Panis, 2000). But it was not flexible to handle this software.
Therefore MlwiN software which has become the under development since late 1980’s was modified at the University of Bristol in UK in order to fulfill that requirement. However, the use of MlwiN for fitting multivariate multilevel models has been challenged by Goldstein, Carpenter and Browne (2014) who concluded that MlwiN was useful if only when fitting the model without imputing for the missing values. However REALCOM software was then came into the field of Statistics and provided the flexibility to impute the missing values in the MLwiN environment.
MLwiN is a modified version of DOS MLn program which uses a command driven interface. MLwiN provides flexibility to fitting very large and complex models using both frequentist and Bayesian estimation along with the missing value imputation in a user friendly interface. Some particular advanced features which are not available in the other packages are included in this software.
In general, data are often collected on multiple correlated outcomes. One major theoretical issue that has dominated the field for many years is modeling the association between risk factors and each outcome in a separate model. It may cause to statistically inefficient since it ignores outcome correlations and common predictor effects (Oman, Kamal and Ambler) (unpublished)
- 上一篇:堪培拉代写Essay 解决组织问题
- 下一篇:堪培拉代写论文:文章和期刊
COPYRIGHT © 2016 EssayMost ALL RIGHTS RESERVED. OUR SERVICE PROVIDED WILL BE USED SOLELY FOR THE PURPOSE OF RESEARCH.网站统计